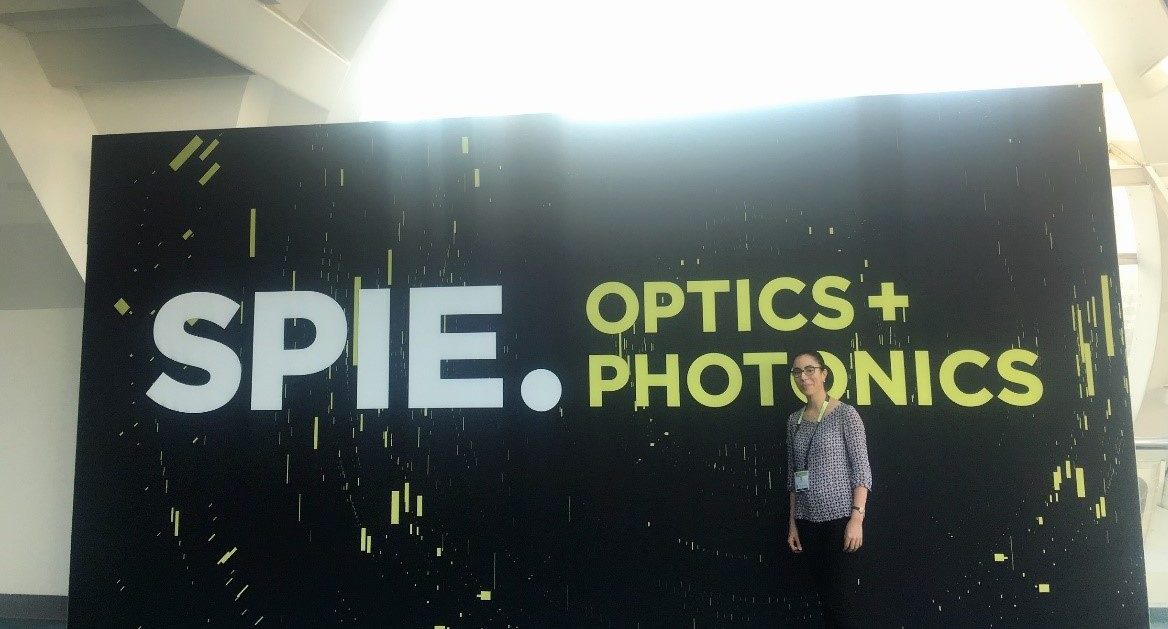
Apizee presented its researches on video QoS enhancement during the SPIE Optical Engineering + Applications conference
Last Updated on May 31, 2021
Even if video conference services providers are perfectly proficient in their interactive video remote collaboration technology in so-called “optimal” network communication situations, they are not yet able to provide the same quality of service (QoS) for communications in locations with limited network coverage.
However, users of remote collaboration tools find themselves regularly in degraded network conditions (limited bandwidth, packet losses, latency …). Whether it is interventions in extreme environments, or mobility situations, such as emergency services vehicles, the need for accurate diagnosis of a distant business expert is real.
In this context, to ensure the quality of video communication in all situations, Apizee is leading a research collaborative project funded by the DGA (French Directorate General of Armaments) and the DGE (French Directorate General for Enterprise) through the RAPID funding program in partnership with the IMT Atlantique Engineering School. To offer an efficient video communication service monitoring , it is essential to identify the representative metrics to measure the QoS and then adapt the service configuration in order to have the best possible quality.
A part of this research was presented last summer in San Diego (CA) during the SPIE Optical Engineering and Applications conference by PhD. Ines Saidi, a researcher at Apizee[1]. The SPIE Optical Engineering and Applications is the premier conference for the latest developments in optical design and engineering, including image and signal processing. In this edition a special interest was given to the applications of image processing by organizing a special session as a topic “System-level optimization in video transmission and communication”. During the event, Ines presented a paper about her researches on video QoS evaluation in partnership with Orange Labs Lannion and INSA Rennes.
The paper focused on real time video quality assessment by considering Machine Learning techniques for modeling the dependencies of different video impairments (such as blur, noise, blocking, flickering, freezing,…etc.) to a global video quality perception using subjective quality feedback.
The investigation lead to the possibility of combining objective single artifact metrics in a video quality assessment model. In fact, these metrics measure from the video the distortion that may be caused by encoding, trans-coding and/or transmission. However, the human perception of the quality does not distinguish between these types of distortion but it gives a global appreciation of the quality. Thus, the objective of the research study was to generate a global model evaluating video quality by training a machine learning on distortion specific metrics.
The obtained model has an accuracy of 63% of correct prediction and detection of bad perceived quality. This result opens new perspectives on the real-time video quality evaluation in all degradation conditions. This methodology is also applicable to the context of WebRTC applications, where the associated QoS statistics such as coding bit rate, frame rate, resolution, packet loss rate and jitter represent accurate metrics to generate a performant video quality assessment model. For that, a huge training database is essential and Apizee has her own API for collecting the QoS statistics for each call handled through their applications which make advanced data mining studies possible with the objective of optimizing the quality of their services.
This study augurs well for the improvement of the quality of service (QoS) on Apizee video communications even on situations of limited network coverage.
[1] Ines Saidi, Ines Saidi, Lu Zhang, Lu Zhang, Vincent Barriac, Vincent Barriac, Olivier Deforges, Olivier Deforges, “Machine Learning approach for global no-reference video quality model generation”, Proc. SPIE 10752, Applications of Digital Image Processing XLI, 1075212 (17 September 2018); doi: 10.1117/12.2320996; //doi.org/10.1117/12.2320996
© 2017 Society of Photo Optical Instrumentation Engineers (SPIE). One print or electronic copy may be made for personal use only. Systematic reproduction and distribution, duplication of any material in this publication for a fee or for commercial purposes, or modification of the contents of the publication are prohibited.